Back in 2017, leading artificial intelligence (AI) thinker and Google Brain project founder Andrew Ng said, “AI is the new electricity” and explained in an interview for Stanford Business School, “Just as electricity transformed almost everything 100 years ago, today I have a hard time thinking of an industry that I don’t think AI will transform in the next several years.” Now, a short six years later, AI has already brought transformative change to the steel industry, allowing manufacturers to seize a competitive edge in an ever-demanding marketplace. Here, AI isn’t a future promise; it’s today’s game-changer. Imagine a factory where machines self-diagnose and auto-schedule their own maintenance – preventing costly breakdowns, minimizing downtime, and optimizing maintenance schedules. That’s predictive maintenance, one of the AI marvels revolutionizing operations and productivity. At SMS group, we have identified strong use cases and successfully implemented the technology in several projects that underline the potential of such applications.
Tap hole blockage prevention
The objective of this development was to address the issue of premature failure of the tap hole, a refractory component through which steel drains in an electric arc furnace (EAF). Conventional efforts, like process optimization, had failed, so it was time to utilize a new approach: In the given customer use case, the tap hole only lasted 20 to 50 heats instead of the industry standard of 100 to 150. The occurrence of blockages, requiring frequent lancing with an oxygen pipe, was a contributing factor to the premature failure.
Our aim was to improve tap hole life and minimize the need for lancing. We developed a predictive analytics system that could assess the risk of tap hole blockages during a heat and enable root cause analysis to identify influential factors.
The dataset consisted of approximately 1,000 signal readings from the EAF across 8,000 heats, with a resolution of every 200 milliseconds. The heats were labeled as ”blocked” or ”free open” based on the presence or absence of blockages at the end of each heat. We deployed machine learning models to cluster tap hole blockage events into distinct segments. These segments revealed key behaviors deviating from the norm, potentially contributing to blockages. We developed a predictive model to quantify the risk of a tap hole blockage by integrating the risks associated with each segment. Afterward, we utilized visualization tools to highlight the behavior of signals associated with the highest-risk segment. This facilitated an in-depth understanding of the contributing factors and supported decision-making for process improvements. SMS groups’ process experts were involved throughout the model development and validation process, to leverage the outputs and drive improvements. For our customer, we were able to increase the rate of flawless tapping from around 80% to over 94%.
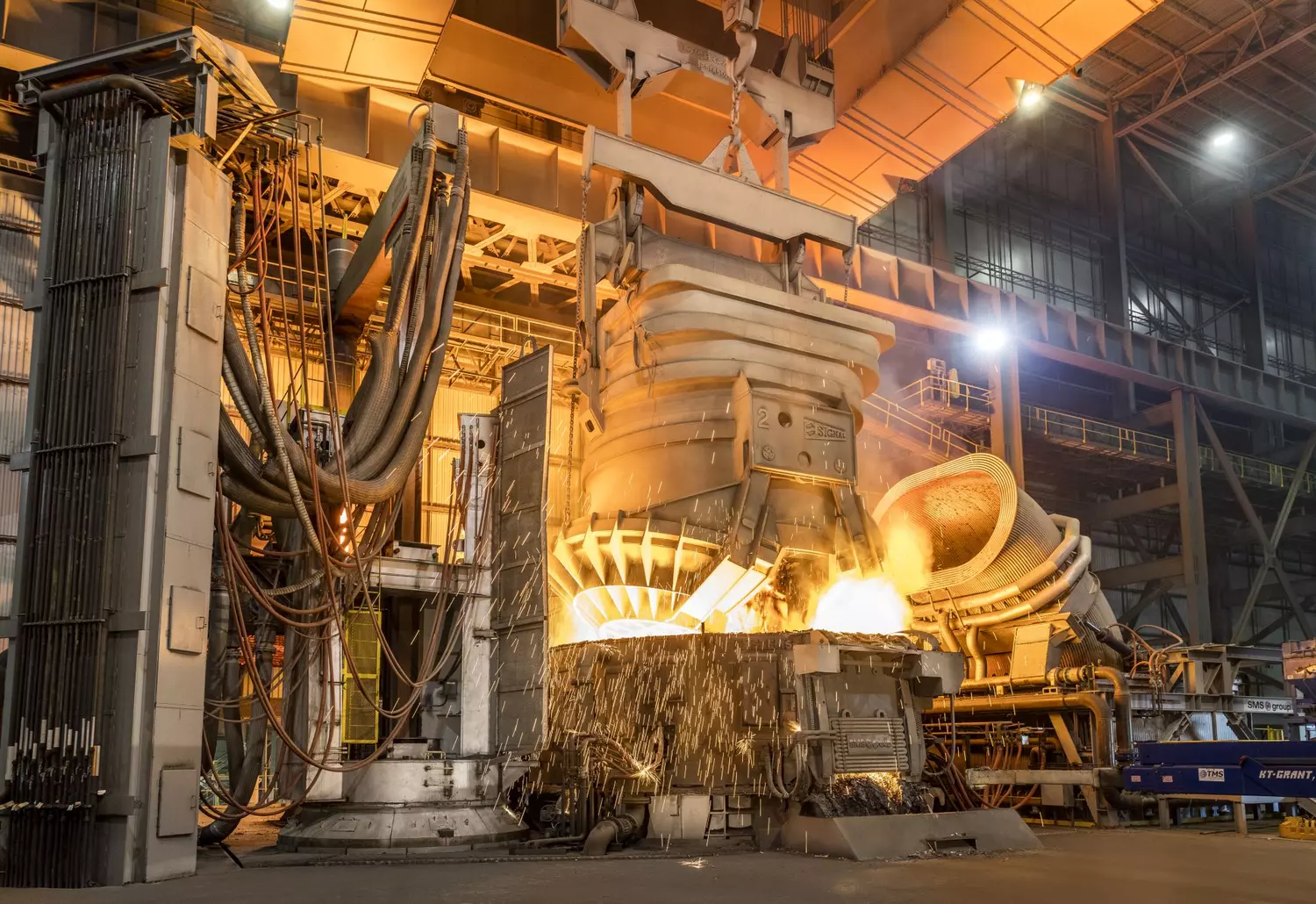
Optimizing EAF efficiency with predictive analytics of cooling panel temperature
The rate of rise (RoR) of the cooling panel temperature of an EAF is a critical factor that impacts a furnace’s performance and lifespan. It refers to how quickly the furnace panel’s temperature increases during operation. If the temperature rises too rapidly, it can lead to premature failure of the refractory lining and damage to the furnace panel, resulting in unplanned downtime and increased maintenance costs. Conventionally, a specific Programmable Logic Controller (PLC) logic is used to prevent overheating on the panels. When a corresponding alarm is triggered, the PLC reduces the electrical energy input into the furnace. Even though this measure prevents increased RoR of the panels, it also results in increased tap-to-tap times (duration between the start of melting the scrap and the tapping of the molten steel) since the power-on time (time of electric energy input) must be prolonged. For our given use case, each heat averaged about one RoR, leading to significant energy costs.
Our objective was to decrease the power-on time of the furnace, thereby increasing throughput and overall operation profitability. Our joint team of digital, automation, and process experts utilized the alarms generated by the PLC to detect sidewall temperature raises and combined that with various sensor inputs.
Based on these data, we developed a machine learning classifier that can predict RoR events before they occur. To achieve this, we developed an advanced predictive analytics system assessing the rate of rise of the EAF panel temperature and detecting potential failure risks. Furthermore, it aimed to facilitate root cause analysis by identifying factors that influence abnormal rate-of-rise patterns. It generates actionable recommendations to prevent such occurrences, aiming to reduce the number of excessively long power-on time heats.
Additionally, we created visual charts that focus on specific areas that are likely to cause an increase in RoR probability. This gives us a clear understanding of what factors contribute to these risks and helps us make better decisions for EAF operations.
The predictive model is able to accurately predict the RoR events with a high degree of accuracy and thus prevents these events. Overall, the case showed that the predictive analytics system can successfully reduce the number of RoR events and improve the productivity of the furnace. In the next project phase, we will decouple the trained model from the PLC logic in order to detach the model from changes in the PLC logic and thus preserve the given prediction quality.