Improved charge-mixes with AI
Over the past few years, the electric arc furnace (EAF) process has become increasingly popular for producing crude steel. Scrap-based EAFs will account for over 50% of crude steel production by 2100. Using scrap instead of virgin materials such as hot briquetted iron or pig iron, carbon emissions during steelmaking can be reduced by approximately 1.67 metric tons per ton of steel. This could ultimately contribute to a decrease in the carbon footprint of steel production.
However, certain types of scrap that are of low quality may contain an uncertain concentration of impurities, which makes it riskier to use them compared to high-quality scrap or virgin materials. These impurities can significantly reduce the quality of the final product, particularly in the case of copper as tramp material. As a result, some operators choose to avoid using excessive amounts of low-quality scrap in their charge mixes and opt for more expensive high-quality scrap and virgin materials. This is especially true when calculating charge mixes manually without the assistance of modern techniques.
To tackle these challenges, machine learning can support us in optimizing the charge mix. Producing one ton of crude steel incurs significant costs primarily due to the raw materials used. To reduce production costs, it is crucial to have a well-planned strategy for utilizing these materials. Low-priced scrap must be used effectively while ensuring the produced crude steel meets production standards. This is especially important regarding undesired tramp elements. The Metallics Optimizer presents a solution that calculates the optimal charge mix by estimating copper predictions using AI techniques in physical process models.
Predicting scrap metal composition
The Metallics Optimizer (MO) is a tool that utilizes artificial intelligence techniques to predict the presence of unwanted tramp elements in scrap metal before it is melted. Based on this prediction, it calculates the most cost-effective composition for the feedstock using optimization algorithms. The application provides valuable information on the chemical makeup of scrap metal, optimizes charge mixes for entire sequences, and reduces production costs while maintaining product quality.
In the process, the Materials Identifier (MI) applies machine learning algorithms to estimate the concentration of all chemical elements in the raw materials. These values are dynamic and serve as charge-mix optimization (CMO) inputs. The dynamic element concentrations are more precise than static values, which cannot account for fluctuations in scrap quality over time due to market availability, supply chain uncertainties, or supplier changes.
The MO application operates in the cloud and stores all necessary data in its database. It can access customer data sources and retrieve the physical characteristics of raw materials from level 2 systems. Information on past and upcoming heats is also pulled from level 3. The ERP system displays the current raw materials and additives inventory and prices. The user interface (UI) offers recommended combinations of raw materials and energy costs for optimal results.
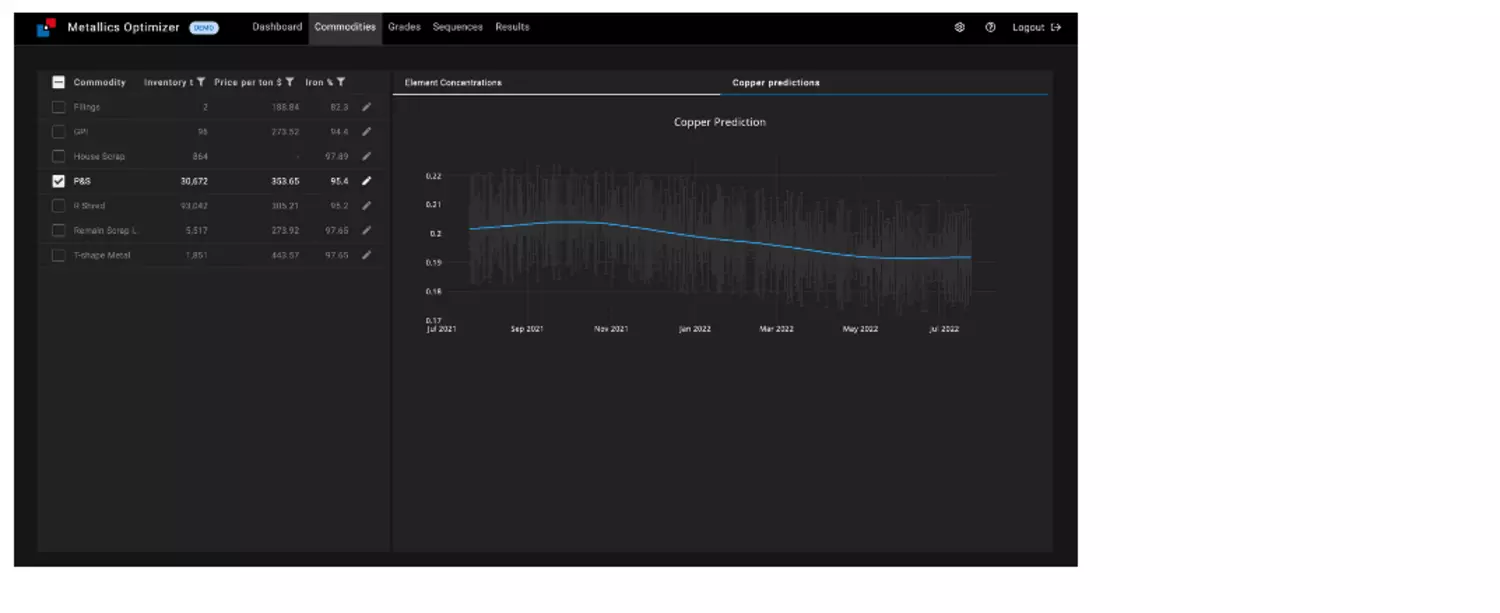
Accurate estimation of copper concentrations
The Materials Identifier uses historical charge-mix recipes, steel analysis, and yield to determine target copper concentrations in commodities. It can be estimated using alternative data if hot heel weight is unavailable. Operators can create new estimates at their preferred intervals, like daily or hourly. The results for estimating copper in two types of scrap samples are displayed in Figure 2. These estimates are shown as non-dimensional values to make them easier to comprehend. The solid blue line indicates the static copper value that the customer used. Home scrap is predicted to have a copper content of only half of what was previously assumed by the static value. This implies that if more of this scrap type is available, it can be utilized for steel grades with lower copper limits.
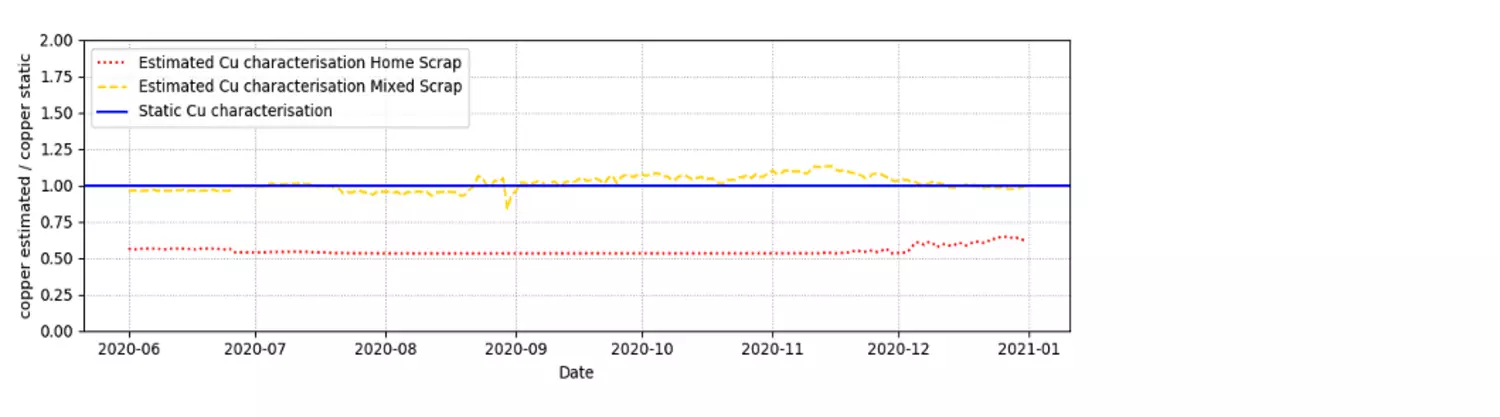
Figure 3 compares past charge mixes to determine the advantages of using MI for producing high-quality steel. The MI's static and estimated copper values are used to perform a mass balance, enabling the calculation of copper content in the final product. This calculated copper content can then be compared to the actual measured copper content, so the ratio between estimated and measured copper content approaches the ideal value of 1.
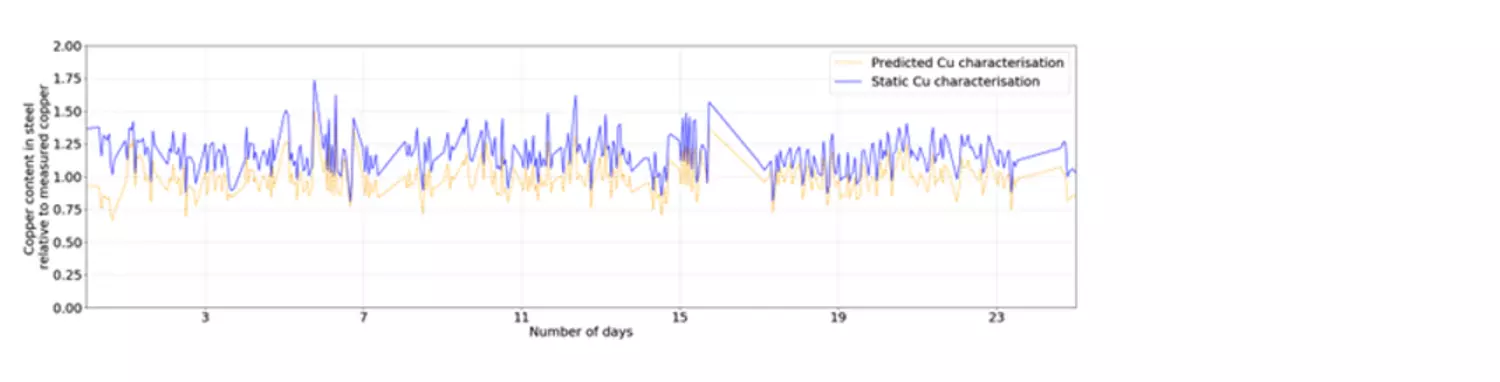
Reducing costs and maintaining production standards
The estimates are sent to the Charge-Mix Optimizer, which calculates charge mixes for any steel grade. The CMO considers expenses and limitations related to raw materials, energy, and technical aspects. It aims to minimize costs through a sequence-based approach while considering transition zones and hot heels. Furnaces with significant hot heels are particularly challenging in this process, as the hot heel of a previous heat influences the next heat. For this reason, transitioning from a heat with a high copper content to a heat with a low copper content can be challenging and sometimes even impossible, even when scrap types with deficient copper concentrations are charged.
The graph in Figure 4 displays the copper concentrations found in the final product. The solid green line represents the copper target, while the dotted blue line represents the copper concentration in the charge mix produced by the customer using the traditional recipe. In certain instances, there is a noticeable difference between the green and blue lines, indicating that the customer is using scrap types that are more costly and have lower copper content than necessary. Using scrap types with higher copper content, which are usually less expensive, the potential for cost savings is visible in the space between the green and blue lines.
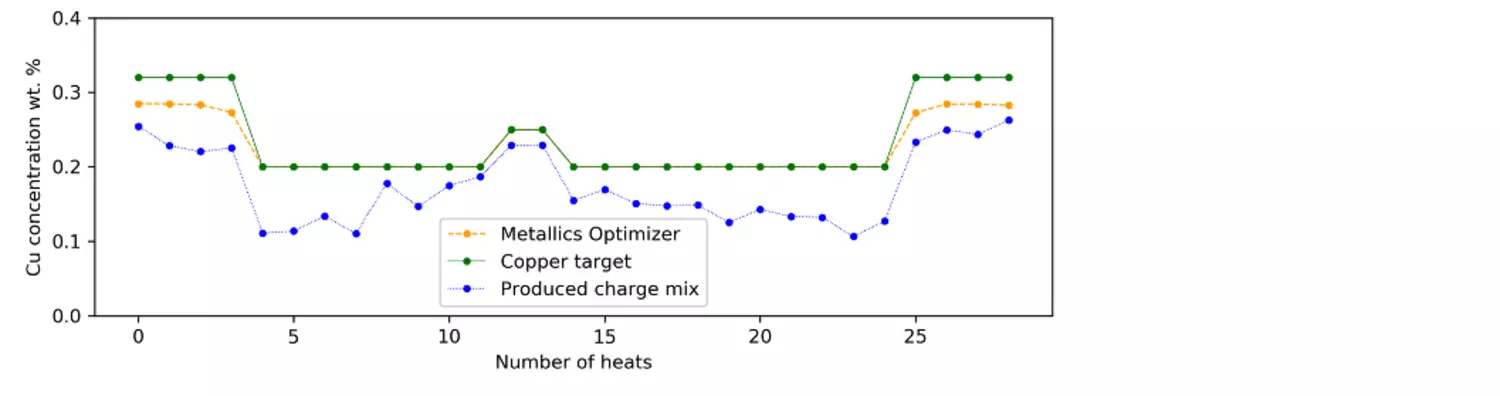
The yellow line shows the CMO's copper mix without a safety margin. The optimizer attains the maximum permissible copper content for a cheaper mix. The first three heats have lower copper, while heats 4 to 5 transition to a lower copper target. The CMO considers the whole sequence and starts with a lower copper concentration in the first three heats, gradually reducing it in heat 4. Copper concentration changes occur in heats 11-14 in a single step, while the last five heats have a 2-step transition to a higher copper target. This may be because the cheapest available scrap types result in a lower copper concentration in the mix than the target.
The Metallics Optimizer proves to be a cost-effective solution for EAF plant operators, resulting in more precise calculations and the ability to use less expensive raw materials with increased copper content.